Post-quantum gravity
The classic conundrum for the last 100 years of wannabe Einsteins is quantizing gravity. I came to the conclusion some time ago that this pursuit is …. aesthetic based at best. Most of the low energy “High Energy” theorists who claim to work on this are numskulls who should be bullycided by experimentalists, but also because it’s fundamentally retarded. As someone put it (maybe Phil Anderson? I can’t locate the quote), there is no more reason for gravity to have a quantum theory any more than there should be a quantum theory of steam engines. They’re both macroscopic phenomena. Gravity in particular is very macroscopic, being much weaker than the other forces of nature.
Something happened last December which as far as I know hasn’t happened in my lifetime: a couple of guys suggested plausible outlines of a theory which resolves the issue and suggested an experiment to put it to the test. Amusingly the main protagonist here, Jonathan Oppenheim, has a bet with my near (and now exceedingly famous: too bad I slept late that year) Gravity professor, Carlo Rovelli, at 5000 to 1 odds that there is no quantum gravity. This is an important enough paper, even the scientific publishers have made it available in cleartext rather than making everyone go through the ridiculous conga dance of looking it up on arxiv or sci-hub. Hopefully this is one of the last gasps of those gate-keeping parasites. Ever notice how science only started to suck with “peer reviewed journal articles?” Some of us certainly have!
Our accepted classical theory of gravity, aka General Relativity, posits that gravity is a sort of geometric phenomenon. Quantizing a geometry is an interesting idea, but you need the continuum to have quantum mechanics and physics involving differential equations in general, so people resort to loops and noodles or whatever. Hence a lot of the trouble with quantizing gravity. This is a pretty good argument in itself that gravity can’t be quantized and is Oppenheim’s point of departure for his ideas.
The theory article is reasonably well written and clearly argued. No hiding behind equation forests here, though there are equation forests. He starts off with “why not go against the consensus that gravity can be quantized and see what happens” to which I say, “just so.” Very Zwicky-like mindset. He gives a history of why people worry about this issue from a theoretical standpoint, and the various paradoxes which come about taking standard views of gravity and quantum mechanics and assuming gravity is non-quantum. His theoretical starting point is something I was dimly aware of called Lindblad operators; a formulation of quantum mechanics where one attempts to model the quantum system in conjunction with its classical environment (and also off diagonal elements). Essentially you model quantum mechanics as a density matrix (which makes more sense than a wave function as it has better classical analogs) coupled to some kind of markovian jumping bean thing which couples the classical environment to the observable variable:
Editorial on this idea: Schroedinger or Heisenberg picture suffer from wave functions diffusing through all of spacetime and collapse of the wave function being sort of added on afterwords. This model is more satisfying in that measurement is baked into the thing. You could argue that it’s an ad-hoc addition, but it gives a more correct answer than Schroedinger picture (which is an ad-hoc Hamilton-Jacobi add-on), even if it is considerably harder to teach in introductory classes. Of course it also kind of does away with absurdities like large scale quantum entanglement if you take it completely seriously. This thing isn’t even unitary!
He beats this thing into various shapes which show that it doesn’t violate other kinds of field theoretical work, making normie field theorists happy, and recovering all the usual stuff we learn in our “modern” physics coursework (aka stuff which is only 75-100 years old). Then he squeezes this idea into the ADM formalism (a sort of toy GR formalism developed to quantize gravity) to develop a “post-Quantum” gravity, which is basically classical gravity with a sort of random noise popcorn machine hooked up to it which is left over from Lindbladian quantum picture.
I’m more or less filtered by his math and the various wankings about renormalization groups and gauge invariance and whatever else makes theorists happy, but my retard view is that he simply takes the Lindblad picture seriously and assumes this is the correct way of looking at quantum mechanics. The paper hasn’t been up long but it already has 37 citations as of my writing this.
The experimental paper gives an overview of the theory, then cites various experiments which bound the decoherence coupling constant -aka the weight of the Markovian popcorn machine term in the Newtonian limit, aka away from relativistic masses where we can use stuff like torsion balances to measure masses and look for excessive noise. They also suggest tests in LIGO, and have an unpublished paper suggesting other ideas for testing the thing.
The paper works through and attempts to dismiss various objections by quantized gravity advocates that a quantum gravity would produce similar outcomes. This is almost certainly where the idea’s attack surface lies. Most of it is impenetrable to me as I’ve never spent more than a few minutes even wondering about such things, but it’s the most obvious theoretical attack on the idea. I guess the other angle of attack is that General Relativity isn’t correct; this only works if you have a theory of gravity involving space-time rather than something like the old Newton’s laws. We know of course that Newton wasn’t quite right in high gravity cases, but the amount of actual experimental evidence for GR is pretty thin; as far as I know it’s all observational. We’re pretty sure it’s OK though.
The proposed experiments to narrow things down: precision measurements of masses using torsion balances as in the Cavendish experiment and other precision gravity measurements. The idea is you should be able to measure this Markovian popcorn thing as noise. How to distinguish this from other kinds of noise? Well, you can’t, but you’d be surprised at how well a good experimentalist can get rid of noise, which would home in on other limits for such a theory. Also one can bound the theory by making large scale (massy) quantum objects and observing their quantum effects for decoherence. These experiments are very cool stuff and ambitious experimental people should be working on them. But Cavendish style experiments (or MEMS doodads): those are things that any sperdo with a machine shop can make and get an answer from. If you’re clever you can move the needle here, and it will look like something a Victorian gentleman could have built (though it will probably have some laser interferometers in it).
One of the fun consequences of all this is it may eventually dispel the phantom of quantum entanglement and force quantum computards to look for productive work, regardless of the excellent Dyakonov argument against such things, which is unrelated to and independent of problems with large scale quantum entanglement. If Lindblad view is the more correct way of looking at quantum mechanics, it’s likely that you can’t couple lots of things together in an entangled state. I’m not sure what the record is for quantum entanglement; I strongly suspect it’s something like 2 otherwise distinct objects. Quantum computers require thousands or millions of classically distinct things to be quantum entangled somehow. Of course there are also other arguments against “macroscopic” entanglement and decoherence. This decoherence thing isn’t something he mentions in the paper, so if he didn’t mean it, don’t blame this statement on him: but that’s more or less what Lindblad is, so I’m pretty sure that’s accurate.
Of course because Oppenheim is a theorist, he has to go after dork matter, and most people are just talking about that. I guess one of the side effects of this idea is it has some MOND-like consequences. It would be interesting to compare his results to the Gerson Otto Ludwig idea I mentioned before. At a glance, Ludwig’s idea produces somewhat different results, but I slept late for that course as I continue lamenting. Ludwig’s scholarship and engagement with the observational data is considerably detailed: there may be a way to simply tease out some different consequences, but this doesn’t even rise to the level of hobby for me, so I leave that to others.
I don’t really have an informed opinion on the topic. If I had to guess or make a bet, Oppenheim isn’t right, in part because he’s a former noodle theorist who came across this idea in some black hole information “paradox” gooning session. Otherwise I’m sure he’s a fine human being and I support his efforts with all my military and naval power. I don’t even understand general relativity well enough (having skipped Carlo’s class) to know much about black holes: it’s just obvious that about 99% of black hole physics papers are unfalsifiable piffle. You can hide a lot of nonsense in singularities. Anyway, this is mere bigotry on my part, I hope he’s onto something. Also because it would be weird if gravity had some Markovian popcorn machine hooked up to it, though it certainly would have escaped notice thus far.
https://www.quantamagazine.org/the-physicist-who-bets-that-gravity-cant-be-quantized-20230710/
https://phys.org/news/2023-12-theory-einstein-gravity-quantum-mechanics.html
More suspect machine learning techniques
Only a few weeks after “Various Marketing Hysterias in Machine Learning” and someone took a big swing at SHAP. Looks like the baseball bat connected, and this widely touted technique is headed for Magic-8 ball land. A few weeks later: another baseball bat to SHAP. I have no doubts that many people will continue using this tool and other such flawed tools. People still write papers about Facebook-Prophet and it’s just a canned GAM model. Even I am a little spooked at how this went: I was going to fiddle with SHAP, but the python-vm contraptions required to make it go in a more civilized statistical environment was too much for me, so I simply made dissatisfied noises at its strident and confused advent (indicative of some kind of baloney), and called it a day. Amusingly my old favorite xgboost now has some kind of SHAP addon in its R package. Mind boggling as xgboost comes with importance sampling which tells you exactly which features are of importance by using the goddamned algorithm in the package!
This little SHAP escapade reminds me of a big one I forgot: t-SNE. This is one I thought should be cool because it’s all metric-spacey, but I could never get to work. I should have taken a hint from the name: t-distributed stochastic nearest neighbor embedding. Later a colleague at Ayasdi (names withheld to protect the innocent) ran some tests on our implementation and effectively proved its uselessness: it’s just a lame random number generator. This turkey was developed in part by neural eminence grise Geoff Hinton -you know, the guy making noise about how autocomplete is going to achieve sentience and kill us all. I think this is why it initially got attention; and it’s not a bad heuristic to look at a new technique when it is touted by talented people. Blind trust in the thing for years though, not so good. At this point there is a veritable cottage industry in writing papers making fun of t-SNE (and its more recent derivative UMAP). There are also passionate defenses of the thing, as far as I can tell, because the results, though basically random, look cool and impress customers. There has always been dimensionality reduction gizmos with visualization like this: Sammon mapping, Multidimensional Scaling (MDS), PacMAP, Kohonen maps, autoencoders, GTMs (PGM version of Kohonen maps), Elastic maps, LDA, Kernel PCA, LLE, MVU, things like IVIS, various kinds of non negative matrix factorization, but also …. PCA. Really you should probably just use PCA or k-means and stop being an algorithm hipster. If you want to rank order them: start with the old ones. Stop if you can’t solve your problem with one of these things which dates after ~2005 or so when the interbutts became how people learn about things: aka through marketing hysterias. I’ve used a number of these things and in real world problems …. I found Kohonen maps to be of marginal hand wavey utility: the t-SNE of its day I guess -almost totally forgotten now; also Kernel PCA, LLE, MDS. I strongly suspect Sammon mapping and MDS are basically the same, and that LDA (Fisher linear discriminants, though Latent Dirichlet seems to work too, it’s out of scope for this one) is probably a better use of my time to fiddle with.
I suspect t-SNE gets the air it does because it looks cool, not because it gives good answers. Rather than being relentlessly marketed, it sold itself because it easily produces cool looking sciency plots (that are meaningless) you can show to customers so you look busy.
Data science, like anything with the word “science” in the name, isn’t scientific, even though it wears the skinsuit of science and has impressive sounding neologisms. It’s sort of pre-scientific, like cooking, except half the techniques and recipes for making things are baloney that only work by accident when they do work.
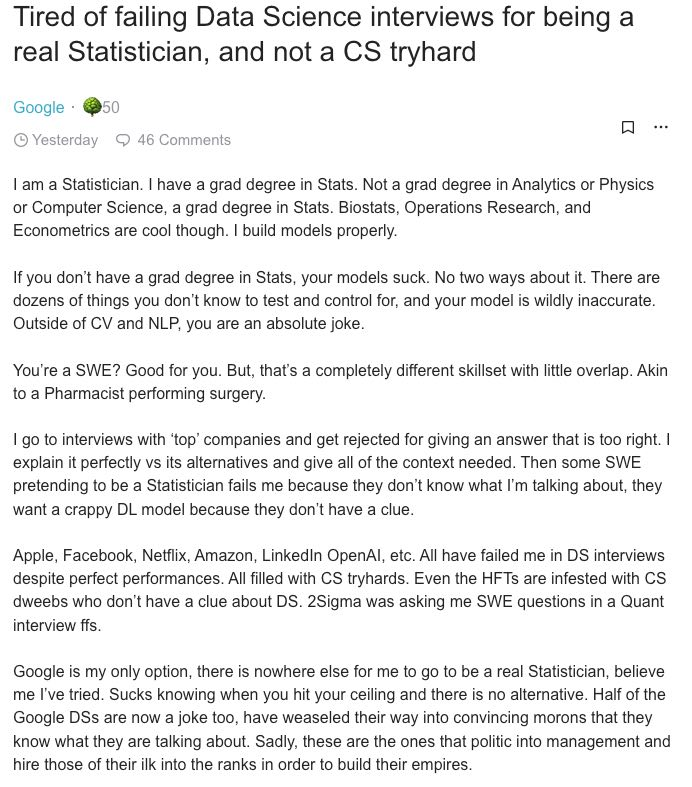
Hilarious autism which I almost agree with
Some older techniques from the first or second generation of “AI” are illustrative as well. Most nerds have read Godel Escher Bach and most will go into transports about it. It’s a fun book, exposing the reader to a lot of interesting ideas about language and mathematical formalisms. Really though, it’s a sort of review of some of the ideas current in “AI” research in his day (Norvig’s PAIP is a more technical review of what actually was being mooted). The idea of “AI” in those days was that one could build fancy parsers and interpreters which would eventually somehow become intelligent; in particular, people were very hot on an idea called Augmented Transition Networks (ATNs) which he gabbles on about endlessly. As I recall the ATN approach fails on inflected languages, meaning if you could make a sentient ATN, this would imply that Russians, Ancient Greeks and Latin speaking Romans are not sentient, which doesn’t seem right to me, Julian Jaynes not withstanding. The idea seems absurd now, and unless you’re using lisp or json relatives (json is a sort of s-expression substitute: thanks Brendan), building a parser is hard and fiddley, so most people never think do it.
Some interesting things came of it; if you use the one-true-editor, M-x doctor will summon one of these things for you. Emacs-doctor/eliza is apparently a fair representation of a Rogerian psychologist: people liked talking to it. It’s only a few lines of code; if you read Winston and Horne (or Paul Graham’s fanfic of W&H) or Norvig you can write your own. People laugh at it now for some reason, but it was taken very seriously back in the day, and it still beats ChatGPT on classic Turing Tests.
Back then it was mooted that this sort of approach could be used to solve problems in general: the “general problem solver” was an early attempt (well documented in PAIP). There’s ancient projects such as Cyc or Soar which still use this approach; expert system shells (ESS -not to be confused with the statistical module for the one true editor) more or less. This is something I fooled around with on a project to give me an excuse for fiddling in Lisp. My conclusion was that an internal wiki was much more useful and easier to maintain than an ESS. These sorts of fancy parsers do have some utility; I understand they’re used to attempt to make sense of things like health insurance terms of service (health insurance companies can’t understand their own terms of service apparently: maybe they should make a wiki), mathematical proof systems, and most famously, these approaches led to technology like Maple, Maxima, Axiom and Mathematica. Amusingly the common lisp versions of the computer algebra ESS idea (Axiom and Maxima) kind of faded out, though Maple and Mathematica both have a sort of common lisp engine inside of them, proving Greenspun’s law, which is particularly apt for computer algebra systems.
Other languages were developed for a sort of iteration on the idea; most famously Prolog. All of these ideas were trotted out with the Fifth Generation Computing project back in the 80s, the last time people thought the AI apocalypse was upon us. As previously mentioned, people didn’t immediately notice that it’s trivial to make an NP-hard query in Prolog, so that idea kind of died when people did realize this. I dunno constraint solvers are pretty neat; it’s too bad there wasn’t a way to constrain it to not make NP-hard queries. Maybe ChatGPT or Google’s retarded thing will help.
yes, let’s ask the latest LLM for how to make Prolog not produce NP-hard constraint solvers
The hype chuckwagon is nothing new. People crave novelty and want to use the latest thing, as if we’re still in a time of great progress, such as when people were doing things like inventing electricity, quantum mechanics, airplanes and diesel engines. Those were real leaps forward, and the type of personality who was attracted to novel things got big successes using the “try all the new things” strategy. Now a days, we have little progress, but we have giant marketing departments putting false things into people’s brains. Nerds seem to have very little in the way of critical faculties to deal with this kind of crap. For myself, I’ve mostly ignored toys like LLMs and concentrate on …. linear regression and counting things. Such humble and non-trendy ideas work remarkably well. If you want to get fancy: regularization is pretty useful and criminally underrated.
Yeah, OK we have genuinely useful stuff like boosting now, also conformal prediction, both of which I think are genuine breakthroughs in ways that LLMs are not. LLMs are like those fiber optic lamps they used to sell to pot heads in the 70s at Spencers Gifts. Made of interesting materials which would eventually be of towering importance for data transmission, but ultimately pretty silly. Most would-be machine learning engineers should probably stick with linear regression for a few years, then the basic machine learning stuff; xgboost, kmeans. Don’t get fancy, you will regret. Definitely don’t waste your career on things you learned about from someone’s human informational centipede. Don’t give me any crap about “how can all those smart people be wrong” -they were wrong about nanotech, fusion, dork matter, autonomous vehicles, string theory and all the other generations of “AI” that didn’t work as well. Active workers in machine learning can’t even get obvious stuff like SHAP and t-SNE (and before these, prophet and SAX and GA and fuzzy logic and case based reasoning) right. Why should you believe modern snake oil merchants on anything?
Current year workers who are fascinated by novelty aren’t going to take it to the bank: you’re best served in current year by being skeptical and understanding the basics. The Renaissance came about not because those great men were novelty seekers: they were men of taste who appreciated the work of the ancients and expanded on them. So it will be in machine learning and statistics. More Marsilio Ficino, less Giordano Bruno.
Pre-spring 2024 books
23 things they don’t tell you about capitalism by Ha-Joon Chang. I found reference to this in one of Aurelien’s excellent blogs. Very good recent economic history, and why things are as fucked up at present now, along with unpleasant (largely forgotten) 2010 era tropes that are better ignored. Chang recognizes the huge problems associated with shareholder value extraction through the PMC, unlimited limited liability companies, the imbecile idea that people are utility optimizing robots, he noticed that Ricardo was a wanker (very based opinion for an economist), and government intervention and protectionism is ridiculously better for most people than “free trade” (which is basically good for oligarch Bezos and the Waltons). He also stands up some shibboleths about “free markets” and salary arbitrage that, as far as I can tell, nobody actually believes, but which makes some kind of dorky economist point (we don’t believe in contemporary economics here: it’s just a shaggy dog story festooned with differential equations in service of whoever is calling the shots). He also lies or at least elides over the truth in a number of places when he’s trying to make a point, which is pretty annoying: I assume he’s a member in good standing with the clerisy and was jockying for position for current year 2010 concerns. Really, this book is “23 things they didn’t tell you in economics indoctrination classes,” but there are more coffee house leftists buying books than economists, so he made the right branding choice. Of course Chang is an economist, and so he should be sewn up in a burlap sack with venomous animals and thrown in a river, but as books on the sins of economists goes, this is a pretty good one and most of his swings at economists connect.
Angle of Attack: Harrison Storms and the Race for the Moon by Mike Gray. Very interesting biography on a largely unsung aeronautics leader in the early jet and space age. Harrison Storms was a bigshot at North American Aviation. NAA built the most successful WW-2 era plane, the P-51 Mustang, and followed it on with the most successful early jet fighter; the F-86, and first supersonic fighter, the F-100. Other solid W’s: the X-15, A-5 Vigilante, XB-70 Valkyrie, the unsung but hugely important SM-64 Navajo, the first US cruise missile, the second stage of the Saturn-V and the Apollo spacecraft. The company was kind of nuked by the Apollo command module fire, despite the fact that it was (according to the telling) a NASA bureaucracy problem rather than a prime contractor problem. Storms took a lot of the blame, and the company was sort of force-merged with one of those ubiquitous 60s conglomerates, Rockwell international (where it was later responsible for the B-1b and Space Shuttle); a company which was most famous for making truck axles and mitre saws -they acquired a water faucet, radio and printing press company around the same time as NAA. Oddly at the time NAA had a ton of cash and Rockwell had more debt than equity, which is why I figure someone must have been encouraging this crap (it might have been dumb luck too).
Industrial conglomerates fascinate me: I think they were encouraged by the government at the time as a way of preserving independent military contractors via diversification. Another weird one: Ling-Temco-Vought. Nuclear cruise missiles, railroads, car rentals, meat packing, golf clubs, steel and pharmaceuticals. None of these businesses have obvious synergy; I guess the idea was risk diversification and perhaps some financial economy of scale. The 1960s funded the creation of many of them via low interest rate takeovers: Gulf Western, Litton, ITT, Textron, General Electric, Teledyne. They all seemed to do a little of everything, including some military/strategic stuff, and often some propaganda/media stuff. This is good as military/strategic stuff is kind of cyclical, and capabilities can be preserved by other businesses. It’s weird the US made so many of these in the 60s, only for most of them to blow up (from excessive debt and creative accounting) in the 70s, and be fragmented or specialized again back in the 80s through 00s. It’s said that index funds (more or less started in the 70s) achieve the same purpose as a conglomerate for investors, and it’s easier to look into one company doing one thing than some weird conglomerate doing dozens of things. The Japanese and Korean Zaibatsus and Chaebols resemble conglomerates, and I suspect many European, Chinese and Indian firms of having similar qualities. One could also look at Berkshire Hathaway as being a conglomerate: perhaps it requires men of a particular genius to make them work. Google seems to be trying and failing to become a technology conglomerate: as far as I can tell all of its subsidiaries with the exception of the investment arms are failures. It might be interesting to look more deeply at the failings and advantages of conglomerates like this.
Back to Harrison Storms: he was one of those guys who got his start in the wind tunnel at CalTech studying with Theodore von Karman. The X-15 and XB-70 were achievements of his. These achievements are easily the equal of anything the Skunkworks put out; hell they’re the equal of everything the Skonkwerks put out. These were enough, but the Apollo spacecraft and 2nd stage Saturn-V kind of put him over the top as one of the all time greats. The earlier achievements are not really documented by this book, which is about Storms bid for the Apollo spacecraft and 2nd stage of the Saturn-V rocket. Really, Storms was a greater engineering manager than any of the other Kelly Johnsons of the world, but as said above; he took the blame for the Apollo-1 fire. Kind of insane from current year perspective; we presently live in an era where managers have no accountability, no matter how incompetent. The book is told from Storms perspective; it’s a result of the author having a long sit down with Storms. Lots of political drama between contractors, various groups in NASA, and heart attacks during periods of extreme stress. Also a sad but glorious ending.
As a point of interest, a man with the same name and more or less same face, who I assume is his son, is a pretty interesting painter.
Burnham’s Celestial Handbook by Robert Burnham jr. I’ve had this 3 book collection on the shelf for a year and a half now (obtained at tremendous personal difficulty). My edition is the 1978 rewrite. This is an encyclopedia of the night sky organized by constellation. I had to look half of them up, as it includes southern constellations I’ve never seen (I’m pretty sure Burnham never saw them either). Burnham was both amateur astronomer and resident astronomer at Lowell observatory. He was decidedly an amateur with no formal training; before he got his observatory job, he worked as a shipping clerk. He was homeless when the survey he was employed at Lowell completed, and barely made a living on book royalties and selling paintings of … cats afterwords. He was such a loner his family didn’t know he was dead until 2 years later. Very tragic figure in some ways, but the man had an inner life the likes of which few great men could aspire to. This series of books is matchless and a must-read for the amateur astronomer; a complete survey of the night sky with poetic descriptions of some of the more awe inspiring sights, vast historical content on the ancient people’s views of the night skies, as well as current-year 1950s-1978 views as to the nature of the things you’ll be looking at. It’s not the type of book you can read from start to finish, though the introductory pages are worth it. I pull it out as bits of the night sky move past my east-facing balcony for what’s going on there, and what Burnham has to say about it.

Blessed spergeloid amateur astronomer
Edison as I knew Him Henry Ford. It’s little known that Ford got his start working in an Edison lab. It’s also underappreciated how great a man Edison was (also, for that matter, Ford). This is a quick survey, I assume some sort of speech (presumably when dedicating the Edison Institute), of their work together over the years. Lots of stuff I didn’t know about Edison; for example, he was home schooled, and he attributed his independent thinking and self-direction to this fact.
Information Theory, Inference, and Learning Algorithms David MacKay. Years ago I read Cover on a plane flight across the US and was mind blown; Information theory is a very different way of thinking about data, and Cover is a uniquely lucid presentation. I bought MacKay at some point and occasionally leafed through it, but recently gave it the ole cover to cover. I had previously found it annoyingly chatty, but it’s actually very good when read in order and it is filled with interesting little problems which actually push the reader towards understanding. Sometimes I think problem sets are just put in there because they’re expected; these are actually helpful. I probably wouldn’t care about information theory, or I might think of it as an annoyingly pretentious field involving boring error correcting codes (they’re not boring) if it weren’t for Cover, which is another incredibly good textbook: odd there are two texts this good in a relatively obscure, though important subject. Not sure information theory is still an active field, though all the machine learning researchers I respect seem to have a very strong background in information theory. Most of it is crap I know already, but I took a passing interest in Turbo codes and relatives, and MacKay has a decent chapter on this, hence this pass through. Reviewing all this stuff on occasion usually pays dividends for me, so this passing curiosity ought to help push the needle. About halfway through: will finish in another month or so. Warning: he has nice lectures but every time he uses chalk it squeaks most distressingly. If he weren’t dead I’d send him fancy Japanese chalk.
The Book of My Life by Girolamo Cardano. Looking around for renaissance autobiographies to avoid reading Benvenuto Cellini for the 3rd or 4th time: this one presented itself. Cardano was a mathematician: his contributions were enormous and largely unsung other than the shitcoin making him harder to google. Some of his math achievements: complex numbers, cubic equations, hypocycloids, negative numbers, binomial coefficients, odds ratios, probability theory in general, imaginary numbers, steganography. He also invented a combination lock, universal joints, gimballed gyroscopes, was a respected doctor who wrote many books (on all kinds of things unrelated to the above subjects). He also had a hard life and a lot of bad luck; his mother tried to abort him and it seemed to get worse after that. Lots of complaints about what seemed like fairly normal misfortunes of his era written from his perspective in his 70s. Lots of interesting coincidences he attributed meaning to. An interesting counterpoint to Cellini’s life: it’s apparently a lot better to be a flamboyant artist than a nerdy physician. It is an odd autobiography in that it more or less consists of lists of things he feels like telling us about; complaints, diet, habits, physical characteristics (of himself), things he wrote, places he visited. On the other hand it is a fucking bummer and he was self loathing and had no idea why he’d be appreciated in future times, so he mostly doesn’t talk about those things. Honestly give it a pass unless you want to read some 15th century nerdoid complain about various vicissitudes of life, or read lists of his friends who you don’t recognize.
Tamburlaine the Great by Christopher Marlowe. I’ve wanted to review some of the other Elizabethan playwrights upon reading myself some Shakespeare last year; Ben Jonson, Thomas Dekker and maybe John Webster are perhaps worth a look, though everyone says Marlowe is the most important. I’ve read his Dr. Faustus in the past (IMO better than Goethe, and well presented in Jan Svankmajer’s puppet movie) and found it as good as anything I’ve read of Shakespeare. I never studied language much, but I am quite fond of Marlowe’s verse in ways I’m not of Shakespeare’s, which often seems crabbed in comparison to Marlowe’s limpid verse. On the other hand, Shakespeare’s dramatic arc and characterization is a lot more interesting than Marlowe’s, at least in Faust (as I recall) and Tamburlaine. Tamburlaine is a lowly bandit in Marlowe’s story who, well, he just kicks ass and takes names to the woe and teeth gnashing of his enemies. It’s sort of like a superhero movie where the hero is psychotic. This was probably great stuff to the people in the day; as I understand things, the excesses of Shakespeare were among the most popular parts. Supposedly a big influence on the Henry VI plays, but I haven’t read ’em yet so I couldn’t say. Was fun to read in any case; modest recommend after reading Faust. I may give his Edward the Second or Jew of Malta (an alleged gore fest and inspiration for Merchant of Venice) a shot; they’re all in the reprint edition I have.
32 comments